Taming Model Sprawl with Domino Model Registry
Tim Law2023-09-12 | 7 min read
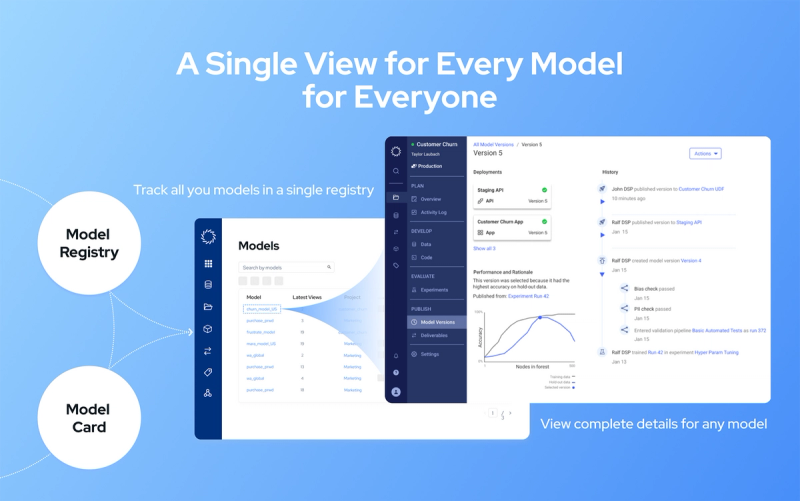
At Rev4, Domino recently announced the launch of Domino Model Sentry, a tightly integrated set of capabilities for building and operating AI responsibly at scale. With Domino Model Sentry, organizations can closely and continuously manage all aspects of AI throughout the entire lifecycle. This article will focus specifically on a core capability of Domino Model Sentry, Model Registry.
The Challenge: Model Sprawl
One of the key AI challenges companies continue to face is “model sprawl,” an inability to quickly and easily discover where models are deployed throughout the organization. In many organizations, different teams have built models on separate platforms, deployed on separate infrastructures, and across various business units. It reflects the outdated, artisanal approach to building and deploying AI. But as AI matures and as companies scale their use of AI, this lack of a centralized view of models introduces risk, inefficiencies, and unnecessary costs. The situation makes it impossible to govern AI in the organization because there is no system of record for model versions, training context, metrics, lineage, and other metadata.
A Single View for Everyone
Domino Model Registry is an indispensable tool for data science projects that systematically tracks and reproduces the training context for all your models through a single pane of glass for any type of model, including traditional predictive models and Generative AI. Model context includes the code, environment, workspace settings, and any datasets or data sources used for each experiment associated with a given model. Beyond the model context, the model registry helps share and discover models, different versions of the model, and associated model cards, which provide model documentation.
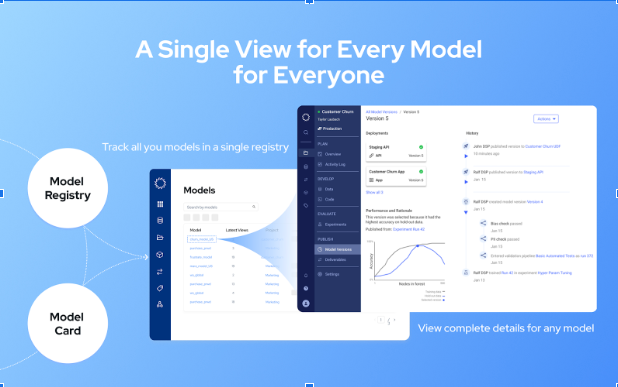
There are many benefits to using a model registry. At the most basic level, a model registry provides a centralized platform to store, manage, and track different versions of models. It helps organize and maintain a repository of models, making it easier for data scientists and engineers to collaborate and share their work. Data scientists can have both Domino users and non-users review their models.
But importantly, it enables data scientists to communicate without any ambiguity by being able to reference each model specifically, which ensures less friction as the model moves through the ML lifecycle. It also leads to higher productivity, enabling unambiguous references to models and their associated lineage. Finally, it also creates documentation of important model artifacts for traceability, reproducibility, and auditability.
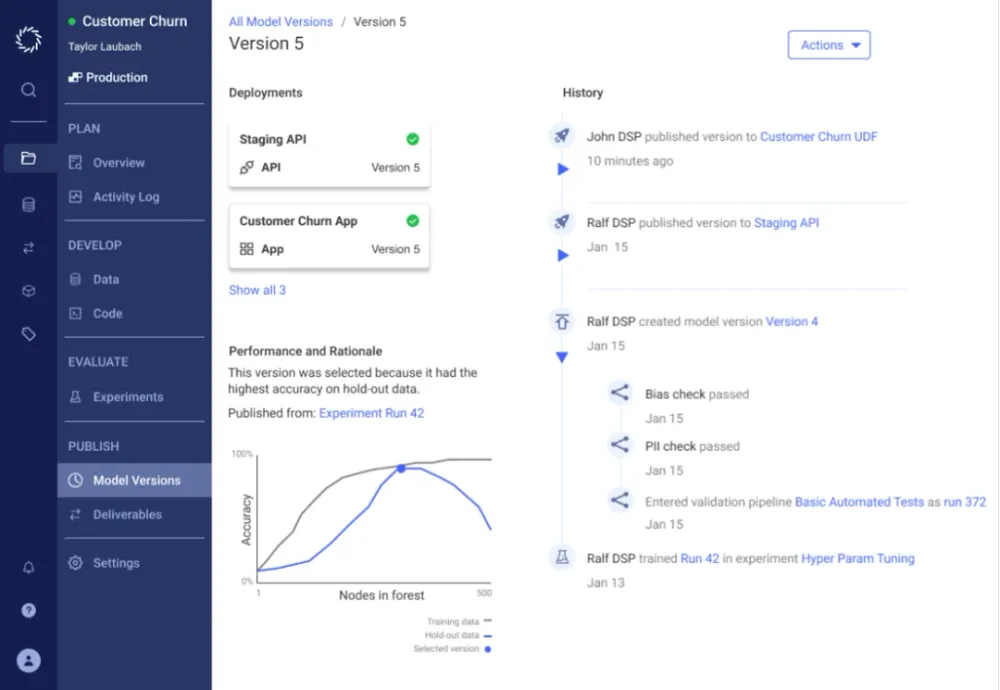
View, Validate, and Govern with Model Cards
Model Registry and Model Cards help govern your models by providing critical information for Domino’s built-in review and approval workflows. Model cards also enable quick model comparisons and facilitate model validation. They provide complete visibility to all essential aspects of model development and performance to be reviewed and approved before being staged for deployment. Importantly, they can alert reviewers to issues that must be addressed before a model can be approved for deployment. Model Registry and Model Cards are essential contributors to the system of record for both pre-production and post-production models.
When publishing your model, you can import model cards from other sources or add markdown content to the model’s description. Custom model cards can combine other resources to provide a single point of truth. For example, you could link to relevant research, dashboards, or external applications that help you evaluate models for fairness and bias.
Scaling AI Confidently
The model registry is a critical way to achieve more scale in your data science organization as your teams produce more and more models. As companies scale AI, it is vital that they can quickly discover, document, and track hundreds or thousands of models at any given time in a single pane of glass. Models are closely tracked, and with a model registry, model versions can confidently be transitioned through each stage in the model review and approval workflow.
Creating Model Transparency
Domino helps you register and manage your models through Model Cards, which are automatically created whenever you publish a model to the registry. Domino uses model cards to track model lineage to contribute to a system of record that helps you evaluate a model’s accuracy, fairness, and compliance.
Model cards document essential information like data science techniques, limitations, performance, fairness considerations, and explainability. Model cards help ensure reproducibility and auditability by automatically tracking metadata, lineage, and downstream usage for each version of your models.
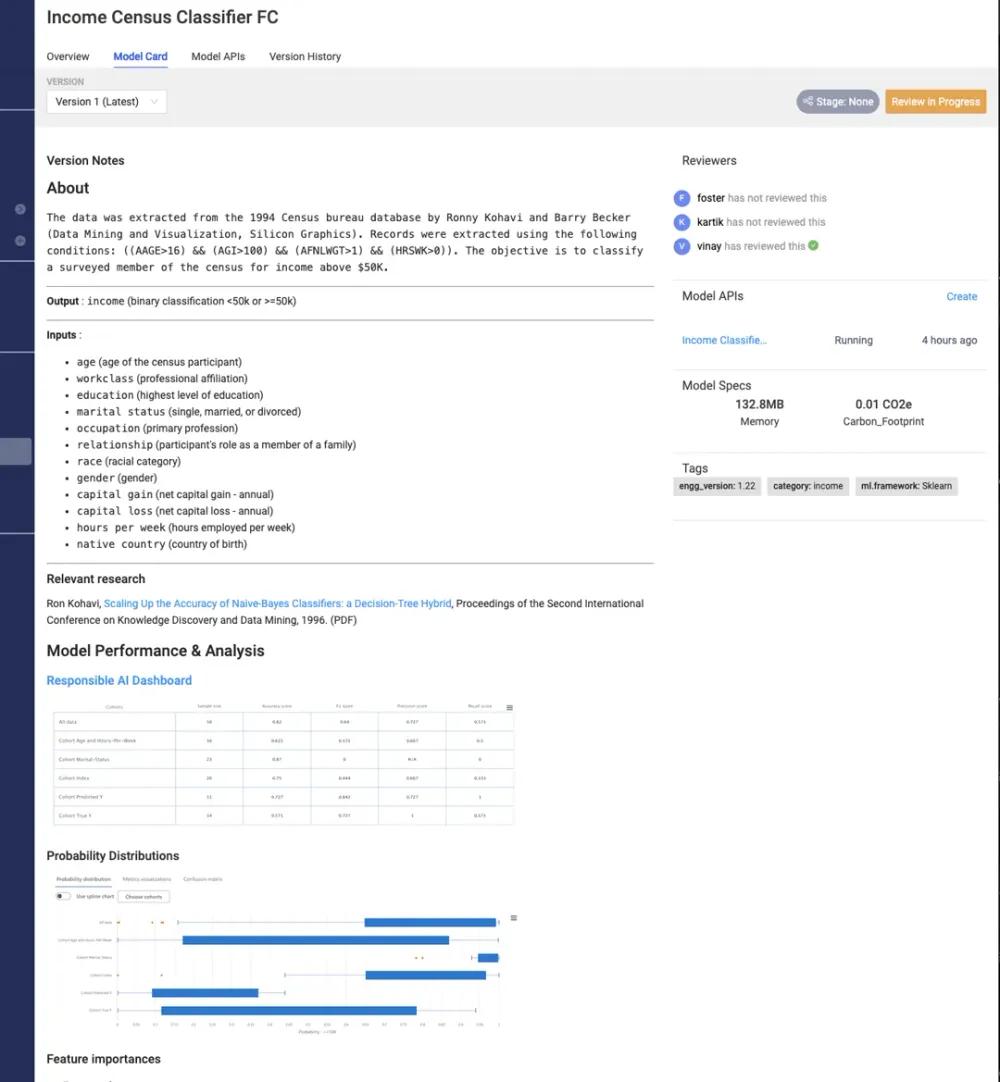
Registering Models is Easy and Secure
Users can register a model in one click while using the Domino web UI after completing an experiment run. But you can also programmatically register your model to the model registry with the MLflow API. You can register models built in Domino or any third-party model and view all your models in a single pane of glass. Use MLFlow tags to add custom attributes to a model or a model version to track additional metadata.
Of course, the Model Registry and Model Cards themselves must be secured and well-governed. Domino uses project-based access controls to determine who can view, manage, and deploy models. Users must authenticate into Domino to use MLflow and Domino APIs for any model task, including model registry actions that a user can perform. But uniquely, users can invite anyone to review their models in view-only, including non-Domino and external users.
Engineering teams benefit from model registries. Because models are documented and stored in a registry, engineering teams can more readily deploy models without spending a great deal of time retraining on a more robust framework, and they can automate much of the retraining. It also, in turn, allows data scientists to focus on creating more models versus having to assist in engineering.
Model Registry helps data science teams be more productive, removes friction from model development and deployment, and helps contribute to the system of record so that projects are transparent and reproducible. But most importantly, it provides transparency and visibility to all the models and AI running throughout your enterprise so you can tame model sprawl.
To learn more about how Domino can help you govern models and tame model sprawl contact us.
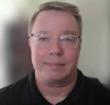
Tim Law, Director of Product Marketing at Domino Data Lab, is an innovative technology marketer with 20+ years of experience in data analytics, AI, and machine learning.
Subscribe to the Domino Newsletter
Receive data science tips and tutorials from leading Data Science leaders, right to your inbox.
By submitting this form you agree to receive communications from Domino related to products and services in accordance with Domino's privacy policy and may opt-out at anytime.