Subject archive for "data," page 9
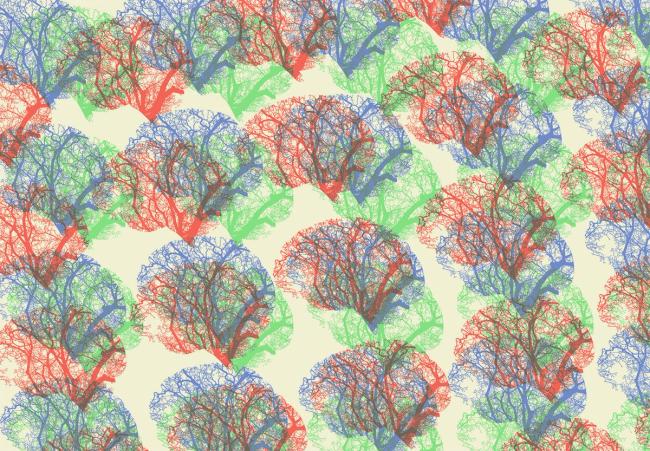
Scaling Machine Learning to Modern Demands
This is a Data Science Popup session by Hristo Spassimirov Paskov, Founder & CEO of ThinkFast.
By Grigoriy34 min read
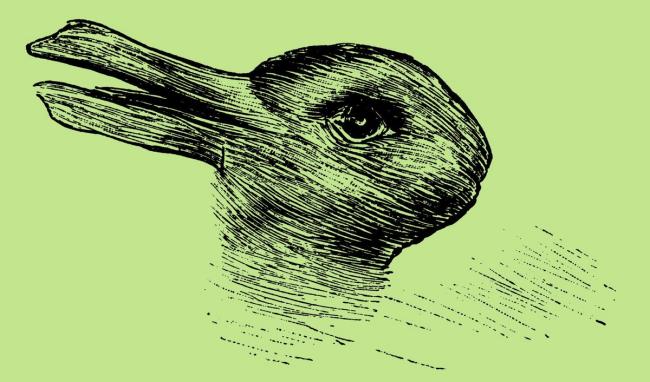
Data Scientists are Analysts are Software Engineers
In this Data Science Popup session, W. Whipple Neely, Director of Data Science at Electronic Arts, explains why data scientists have responsibilities beyond just data science.
By Grigoriy29 min read
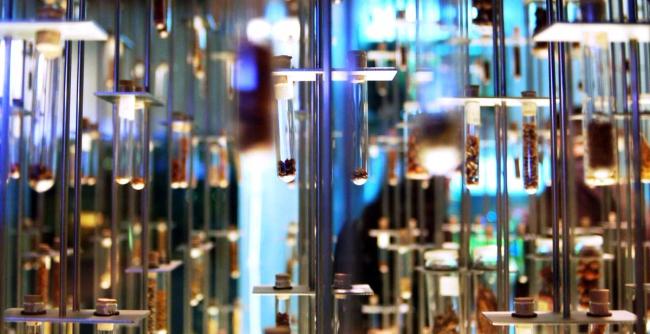
Horizontal Scaling for Parallel Experimentation
The amount of time data scientists spend waiting for experiment results is the difference between making incremental improvements and making significant advances. With parallel experimentation, data scientists can run more experiments faster, leaving more time to try novel and unorthodox approaches—the kind that leads to exponential improvements and discoveries.
By Eduardo Ariño de la Rubia6 min read
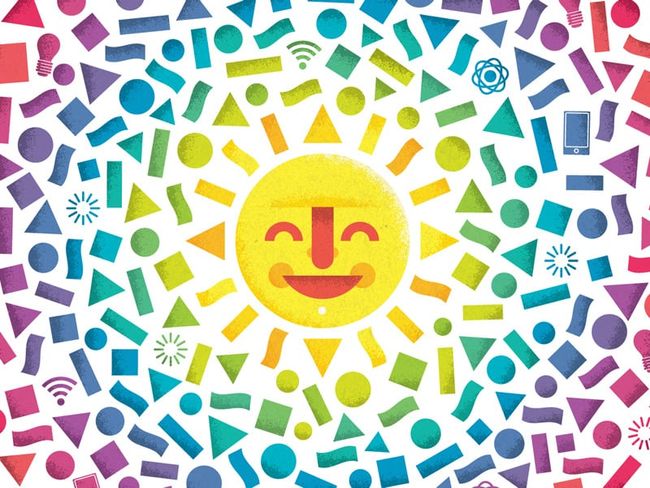
Fighting Child Exploitation with Data Science
Every day, 100,000 new escort ads are posted online. That is according to Thorn, a nonprofit that fights child sexual exploitation through technology innovation. Other studies by Thorn on the underage sex trafficking situations of survivors have shown that 63% of them had been advertised online at some point. The massive online commercial sex market is extremely difficult to fight, which has inspired the start of an annual hackathon to bring cross-industry experts together to work on child safety.
By Kimberly Shenk4 min read
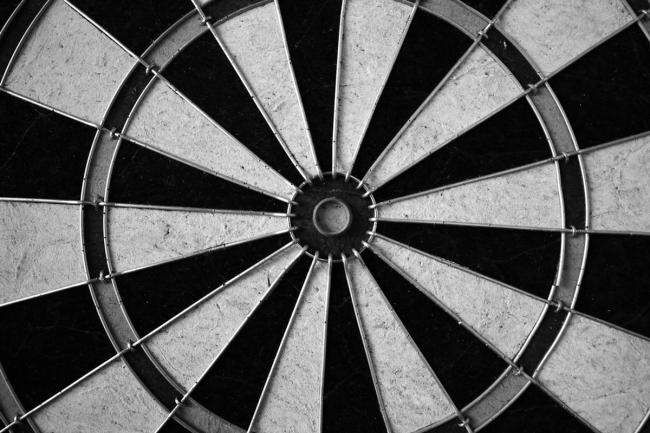
Succeeding with Alternative Data and Machine Learning
Perhaps the biggest insight in feature engineering in the last decade was the realization that you could predict a person's behavior by understanding the behavior of their social network. This use of non-traditional social data has driven a significant amount of economic growth as it revolutionized the accuracy and applicability of models.
By Grigoriy50 min read
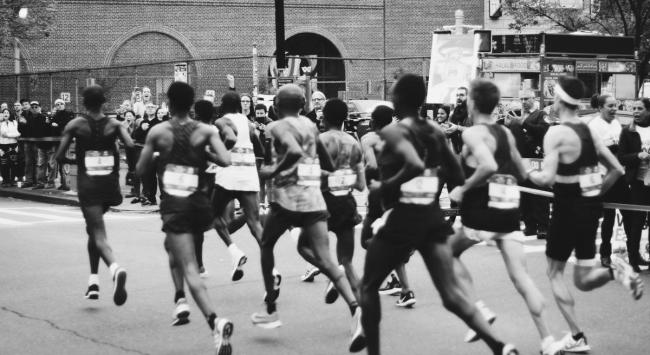
Benchmarking Predictive Models
It's been said that debugging is harder than programming. If we, as data scientists, are developing models ("programming") at the limits of our understanding, then we're probably not smart enough to validate those models (“debug”) effectively.
By Eduardo Ariño de la Rubia13 min read
Subscribe to the Domino Newsletter
Receive data science tips and tutorials from leading Data Science leaders, right to your inbox.
By submitting this form you agree to receive communications from Domino related to products and services in accordance with Domino's privacy policy and may opt-out at anytime.