Subject archive for "model-management," page 13
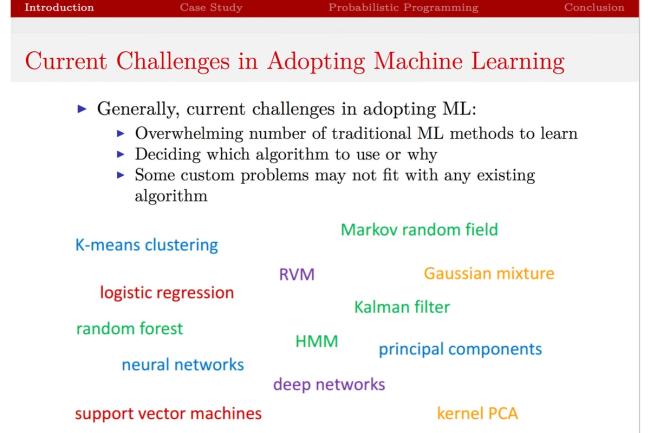
Model-Based Machine Learning and Probabilistic Programming in RStan
In this recorded webcast, Daniel Emaasit introduces model-based machine learning and related concepts, practices and tools such as Bayes' Theorem, probabilistic programming, and RStan.
By Daniel Chalef1 min read
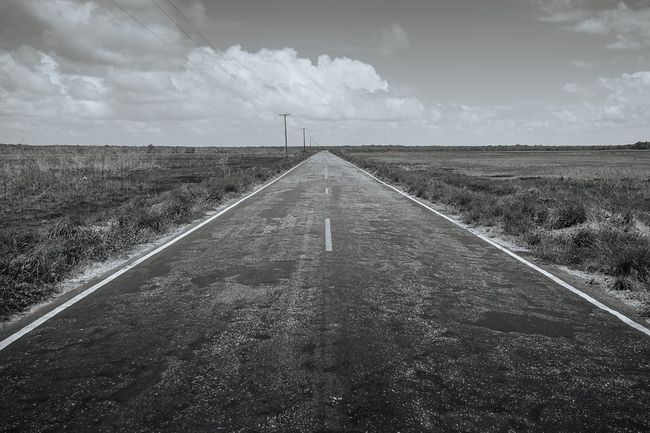
Providing Digital Provenance: from Modeling through Production
At last week's useR! R User conference, I spoke on digital provenance, the importance of reproducible research, and how Domino has solved many of the challenges faced by data scientists when attempting this best practice. More on the topic, and a recording of the talk, below.
By Eduardo Ariño de la Rubia1 min read
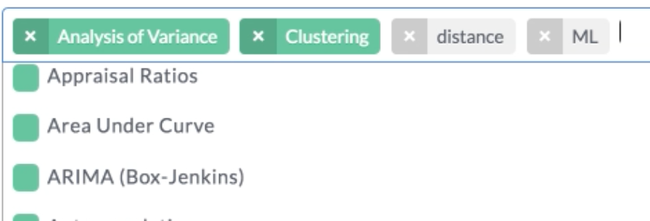
Better Knowledge Management for Data Science Teams
We’re excited to announce a set of big new features that make it easier for you to find and reuse past data science work in your team and organization. We’re calling these features “Discoverability,” and they encompass powerful ways to search, tag, and get recommendations about relevant analytical work.
By Nick Elprin7 min read
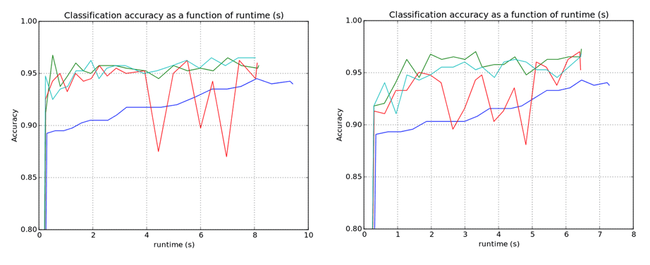
“Unit testing” for data science
An interesting topic we often hear data science organizations talk about is “unit testing.” It’s a longstanding best practice for building software, but it’s not quite clear what it really means for quantitative research work — let alone how to implement such a practice. This post describes our view on this topic, and how we’ve designed Domino to facilitate what we see as relevant best practices.
By Nick Elprin5 min read
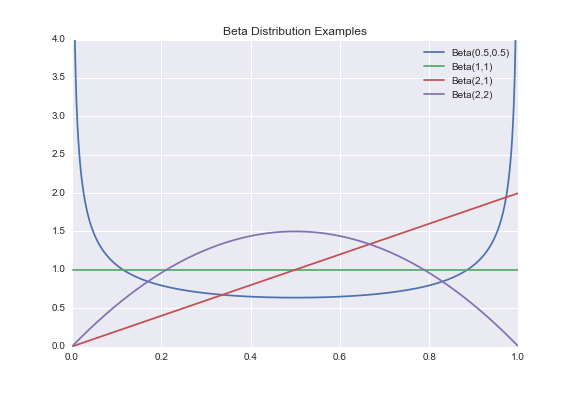
A/B Testing with Hierarchical Models in Python
In this post, I discuss a method for A/B testing using Beta-Binomial Hierarchical models to correct for a common pitfall when testing multiple hypotheses. I will compare it to the classical method of using Bernoulli models for p-value, and cover other advantages hierarchical models have over the classical model.
By Manojit Nandi23 min read
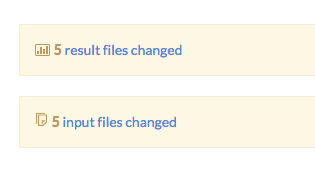
Faster model tuning and experimentation
Domino provides a great way to iterate on analytical models by letting you run many experiments in parallel on powerful hardware and automatically track their results. Recently we added two powerful new features to make that workflow even easier: Run Comparisons and Diagnostic Statistics.
By Nick Elprin4 min read
Subscribe to the Domino Newsletter
Receive data science tips and tutorials from leading Data Science leaders, right to your inbox.
By submitting this form you agree to receive communications from Domino related to products and services in accordance with Domino's privacy policy and may opt-out at anytime.