Featured Success Stories
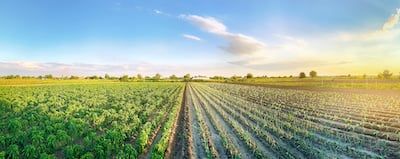
Bayer
Bayer is able to test more seed variants, produce more seed with less land, and serve customers better.
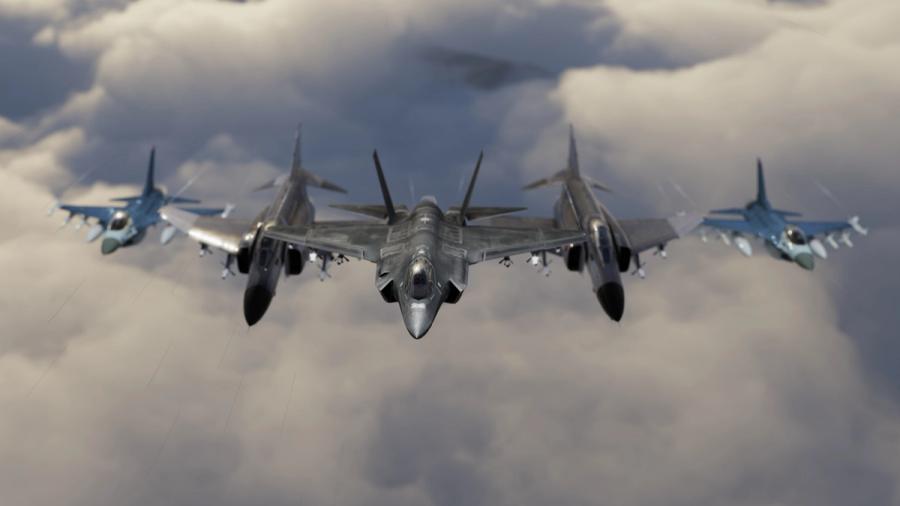
Lockheed Martin
Lockheed’s scalable approach to managing artificial intelligence yields $20 million in value each year and delivers new innovations faster.
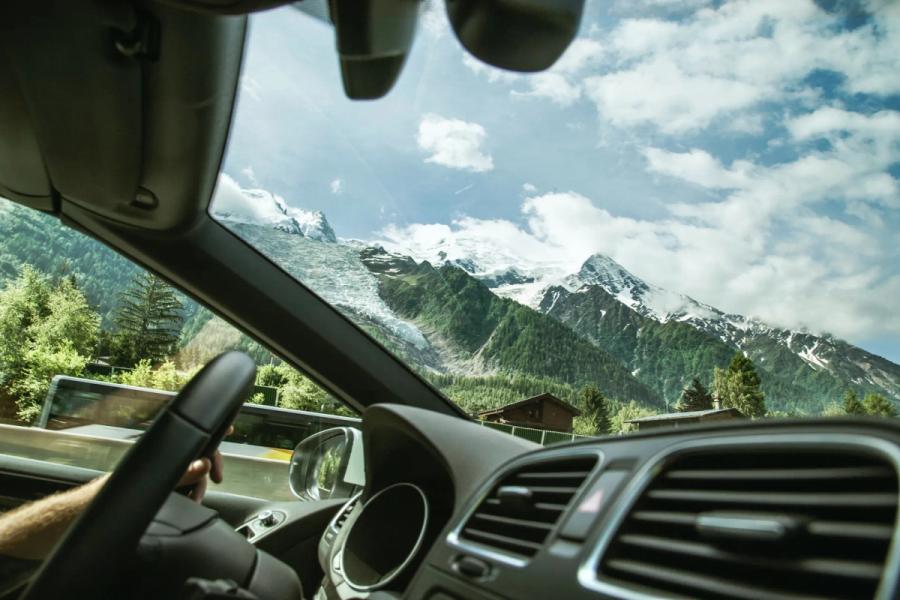
Allstate
Allstate delivers a faster, more seamless claims process with the ability to test new ideas more easily and develop models faster.
Experience breakthroughs in every industry
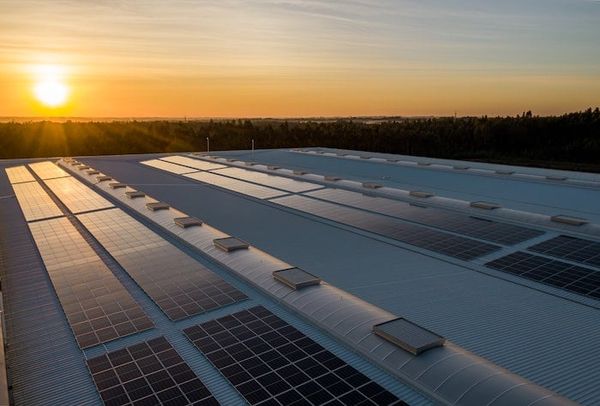
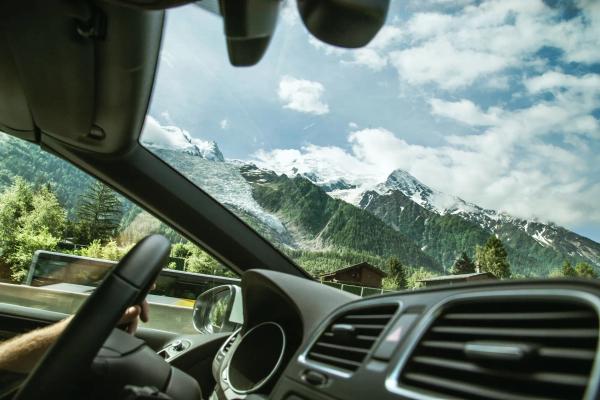
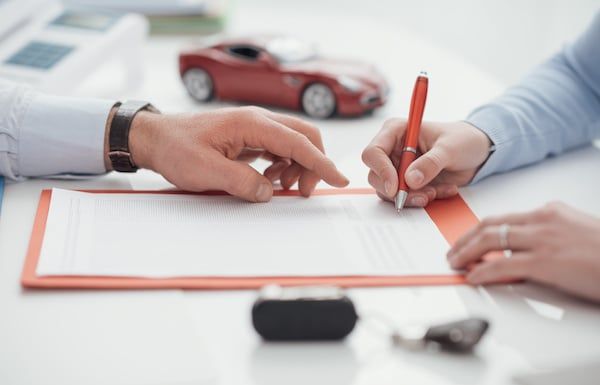
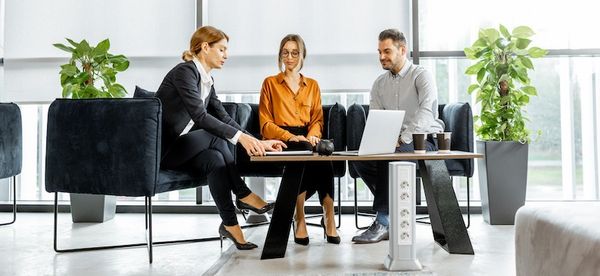
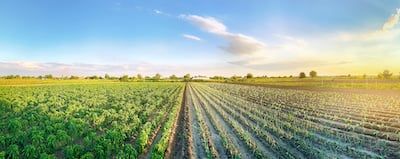
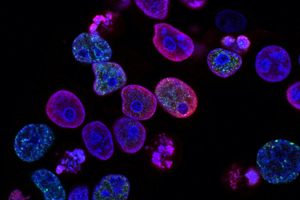
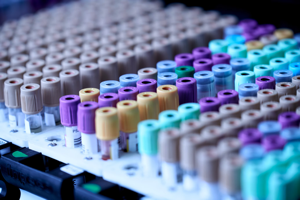
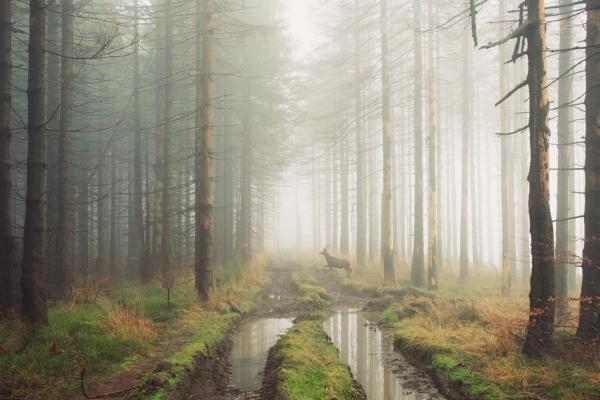
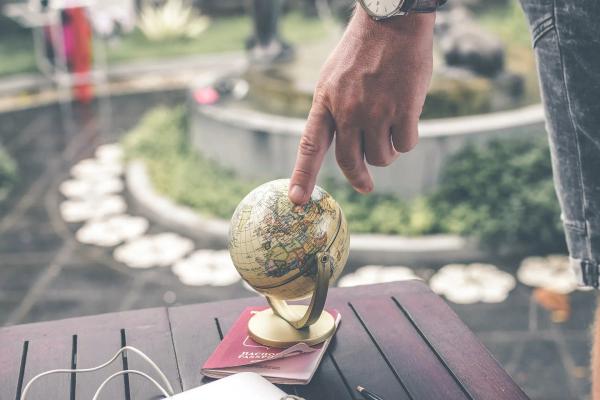