episode 45
Change Management Strategies for Data & Analytics Transformations
Data Science Leaders | 38:52 | April 06, 2022
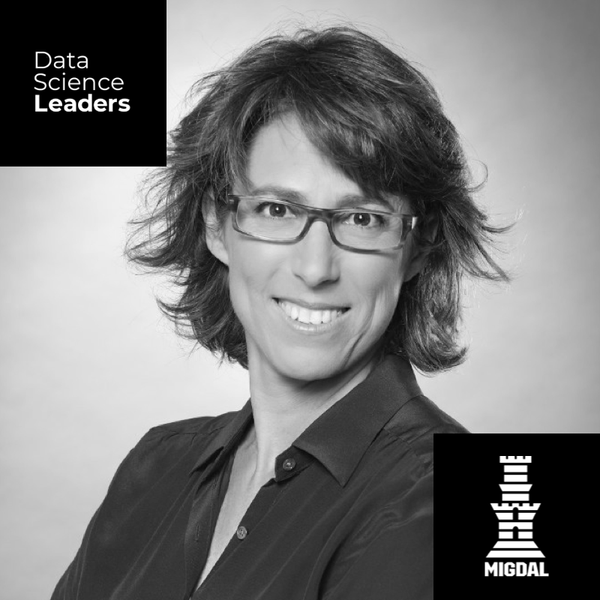
Listen how you want
Get new episodes in your inbox
Large enterprises will always have some internal groups that are more change-averse than others. But progress often necessitates change, and how well you navigate the change management process can make or break your success as a leader.
Michal Levitzky is the Head of Data & Analytics (CDO) at Migdal Group, a leading insurance and finance company in Israel. Michal has spearheaded the introduction of data and analytics functions at multiple organizations, and she knows a thing or two about negotiating the complexities of change management during analytics transformations.
In this episode, Michal shares her advice for AI leaders driving meaningful change at their own companies. Plus she details her philosophy on structuring data and analytics teams for maximum efficiency and collaboration.
We discuss:
- Using experience in fields like accounting as building blocks for leadership in data science
- Change management during model-driven transformations
- A structure to enable BI and data science functions to better support each other
Popular episodes
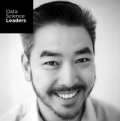
James Cham
Partner, Bloomberg Beta
EPISODE 71April 11, 2024
Unlocking the disruptive potential of generative AI: a VC perspective
Listen Now | 28:49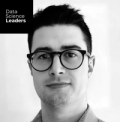
Volodimir Olexiouk
Director of Scientific Engagement and Data Science Team Lead, BioLizard
EPISODE 70March 28, 2024
Overcoming the data challenges of AI-driven drug discovery
Listen Now | 36:49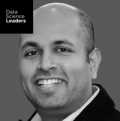
Rahul Todkar
Head of Data and AI, Tripadvisor