Five Tips on Leading Your Data Science Function to MLOps Excellence
Glenn Hofmann2021-08-05 | 9 min read
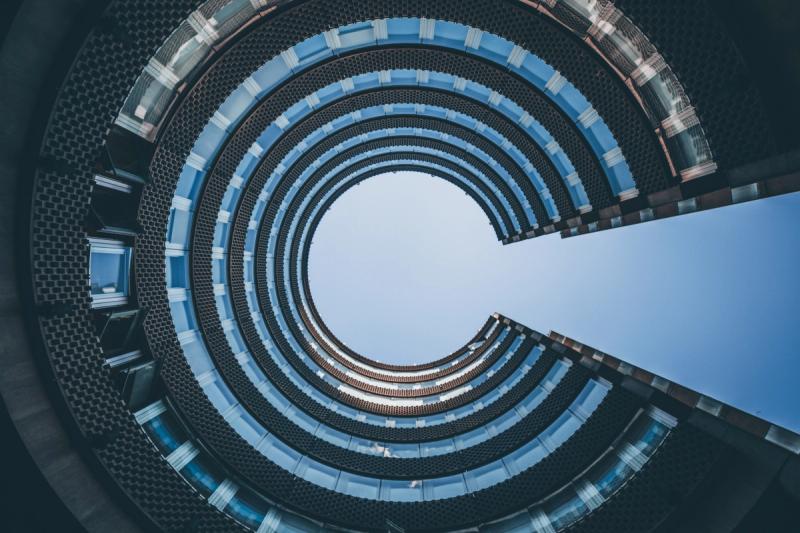
Editor’s note: This is part of a series of articles sharing best practices from companies developing an enterprise data science strategy. Some articles will include information about their use of Domino.
During the last 15 years of my post-academic career, I have had the privilege of developing data science teams for companies in the finance and insurance sectors. I was recently asked to reflect on what actions contribute to making a data science team successfully integrated into an organization. Below are five tips that I offer to anyone embarking on the journey of building out an enterprise data science function, and leading a team to ML Ops excellence.
Hire a diverse skill set
When I joined New York Life five years ago, I was tasked to grow a seven member data science team to one that would function as the enterprise-wide, centralized data science hub and provide artificial intelligence solutions with new levels of service, expertise, and efficiency across the company.
Today, the Center for Data Science and Artificial Intelligence (CDSAi), now a 50-person team, features the diverse skill sets required to successfully deliver data science and artificial intelligence expertise, solutions, and education across our Fortune 100 life insurance company. CDSAi includes not only data scientists, but also machine learning engineers, data engineers, project and product managers, operations and change management leads, development managers for building external partnerships, and data science community leaders.
Our data scientists are involved in every aspect of the model development life cycle. They are supported by project managers and ML Ops engineers who are responsible for model deployment and our various technology platforms. Our change managers, product managers, and development managers work hand-in-hand to ideate and integrate data science across new business partners.
Promote transparency and data science education
Much has been said on the importance of demystifying data science and that has been true of every organization that I’ve been associated with. The more informed and knowledgeable our business partners are, the more excited they are to incorporate data science into their decision-making processes. To that end, we have created multiple ways for the company to learn about data science and become involved.
Thanks to strong support within New York Life, we have successfully established an inclusive data science community which reaches across all areas, occupations, ranks and levels of experience with data science through a variety of programs. These include:
- Monthly “Lunch & Learns” on projects, methodologies, and data
- Annual data science expo
- Data science forums
- External guest speakers
Through these events, our internal data scientists continue to learn from each other and interested employees across the company are exposed to applications of data science in different business areas.
Furthermore, through our Data Science Academy, we have created opportunities for all employees at New York Life to learn about data science at the level where they are most comfortable. This includes both technical and non-technical certification pathways as well as article and video series. We have hosted a range of workshops that cover topics from how to get started with Python to how our senior executives can make the most of our data science capabilities.
Through these events, learning opportunities, and New York Life’s intranet, we have raised the level of understanding of what data science is and highlighted the tremendous value that it can provide. This helps our relationships with our existing business partners, potential new ones, and with other stakeholders too.
Connect frequently and meaningfully
Continuing on the theme that the more knowledgeable your business partners are, the more excited they are to embrace data science into their decision-making processes, the CDSAi team is highly encouraged to connect with our business partners frequently.
For our data scientists, this means meeting on a weekly or bi-weekly basis with stakeholders. There may not always be a significant update, but in those meetings we continue to learn nuances of the business as well as pain points and they learn about our process. It’s mutually beneficial, and on a personal level, enjoyable and rewarding. Other team members are involved in these regularly scheduled meetings too. Project milestones are celebrated together. Working virtually has certainly not stopped us from acknowledging all the important moments in a project either.
Beyond these meetings, our team members interact with others around the company regularly as well. This helps in learning more of the business and how we can help, but also, it’s a fulfilling part of the job to get to know others on a meaningful level. Finding the points where we connect with our colleagues is important both for personal engagement and for ensuring a smoothly run project.
Align with all stakeholders
We believe it’s vital to ensure we’ve effectively aligned our efforts with all stakeholders including:
- Business Leaders: We frequently speak with business leaders within our Sales, Marketing, Finance, Product, Service and other business teams to discuss opportunities and ensure we’re aligned. CDSAi managers are very much aligned with their counterparts in the business units we serve.
- Technology: We need to be nimble regarding technology, especially as we rapidly bring new platforms, libraries, and tooling into our data science ecosystem to assist our team in driving innovation. Good communication with our partners in Technology is critical for this. With every request, we share why we need a specific technology and how it moves us forward from both a technology and business perspective. Additionally, our data engineers actively work with Technology to identify data priorities and how data will flow into analytics development. The CDSAi team then handles the “last mile” of data processing to get the data into our models.
- Governance Teams: As a company, we have extensive governance processes. There are a variety of governance teams we work with for larger projects. As a result, in all our project plans, we have processes to ensure our teams start these conversations months before we need a decision so we can ensure we’re addressing any requirements early in development.
Invest in technology infrastructure
We invest in infrastructure that can help accelerate model development and deployment. For example, we’ve created an environment for computing using Python and R stacks and model development. More recently, we’ve created an infrastructure for model deployment with the help of the Domino platform. We use Domino to eliminate manual efforts to move a model into production, which requires a lot of quality assurance (QA) work to get it right. Now, with the Domino platform and Kubernetes cluster underlying it, we’ve eliminated months of recoding work and can bring Python and R code directly to production. Our models can now be accessed from any production platform in the company via an API. Implementing, running, and managing the Domino platform and deploying models is very much a collaboration between CDSAi and our Technology team.
Final reflection
I’m incredibly proud of the impact CDSAi has had in helping diverse teams at New York Life make model-driven decisions. Functions across Sales, Marketing, Underwriting, and Agency, to name a few, are employing data science to automate critical processes and improve decision making so they can better serve our customers. The team has built a strong foundation — spanning people, processes, and technologies — and has been crucial in helping deliver this impact efficiently and collaboratively.
As we further scale the data science capability across New York Life, efforts remain underway on many fronts. For example, one recent effort is the work to enhance our data streams and create a feature store that documents and institutionalizes knowledge about the best features to use for particular models. Feature engineering is a time-consuming process, and this work will enable our teams to continually build on each other’s knowledge and solve complex business problems faster.
The five tips above are from my experience and the journey certainly continues.
Glenn Hofmann is currently the Chief Analytics Officer at New York Life Insurance Company (NYL), a Fortune 100 firm, where he combines extensive knowledge in applied statistics, analytics and data infrastructure with deep expertise in building and leading large teams of data- and analytics-focused professionals from the ground up to influence strategy as well as transactional decisions through data science-driven innovation. Glenn has previously held leadership positions at Allstate, TransUnion, and Verisk Analytics.
RELATED TAGS
Subscribe to the Domino Newsletter
Receive data science tips and tutorials from leading Data Science leaders, right to your inbox.
By submitting this form you agree to receive communications from Domino related to products and services in accordance with Domino's privacy policy and may opt-out at anytime.