Blog archive, page 6
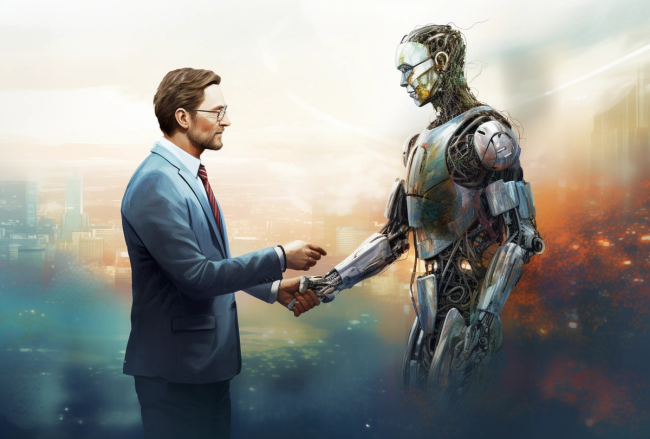
Generative AI Must Be Responsible AI. What You Need to Know.
Companies diving head-first into Generative AI must consider the risks it brings. Domino’s guide shows you how to harness GenAI responsibly. Download now!
By Yuval Zukerman3 min read
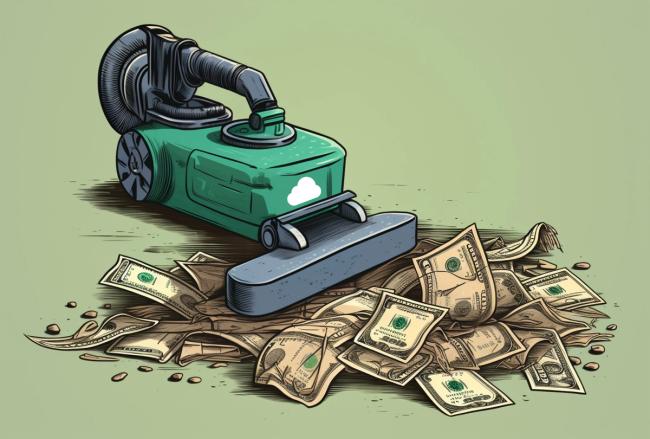
AI Costs Keeping You Up at Night? It's time for Domino
AI costs rise with data & infrastructure needs. Domino's guide offers advice on cost controls, productivity, governance & risk - to save you money.
By Yuval Zukerman4 min read
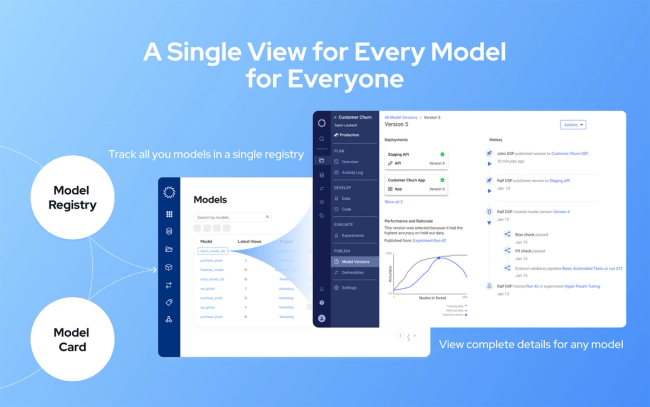
Taming Model Sprawl with Domino Model Registry
At Rev4, Domino recently announced the launch of Domino Model Sentry, a tightly integrated set of capabilities for building and operating AI responsibly at scale. With Domino Model Sentry, organizations can closely and continuously manage all aspects of AI throughout the entire lifecycle. This article will focus specifically on a core capability of Domino Model Sentry, Model Registry.
By Tim Law7 min read
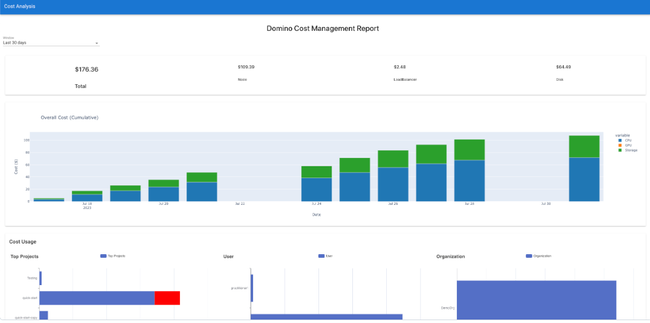
Domino’s New Cost Governance Capabilities for AI Drive Accountability, Visibility & Savings
Controlling and tracking AI, data science and related compute costs are often manual and error prone, and require tagging specific infrastructure to distributed IT workloads. Domino.ai provides the governance guardrails necessary to reduce and control infrastructure costs, including expensive high-performance compute (e.g. GPUs) resources.
By Nikhil Jethava6 min read
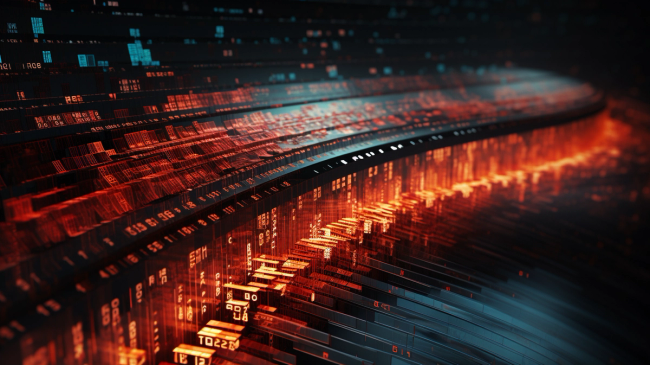
Crossing the Frontier: LLM Inference on Domino
Generative AI transforms industries, but LLM deployment is tough. See how Domino simplifies LLM hosting & inference.
By Subir Mansukhani10 min read
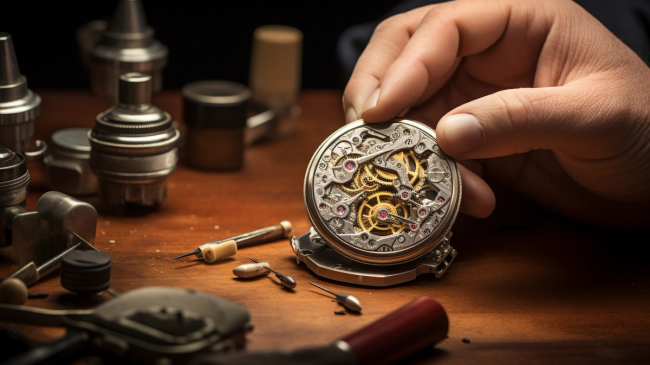
Breaking Generative AI Barriers with Efficient Fine-Tuning Techniques
This blog post explores the challenges of fine-tuning large language models (LLMs) and introduces resource-optimized and parameter-efficient techniques such as quantization, LoRA, and Zero Redundancy Optimization (ZeRO). By fine-tuning Falcon-7b, Falcon-40b, and GPTJ-6b, we demonstrate how these techniques offer improved performance, cost-effectiveness, and resource optimization in LLM fine-tuning. The blog post also discusses the future of fine-tuning and its potential for unlocking new possibilities in enterprise AI applications.
By Subir Mansukhani9 min read
Subscribe to the Domino Newsletter
Receive data science tips and tutorials from leading Data Science leaders, right to your inbox.
By submitting this form you agree to receive communications from Domino related to products and services in accordance with Domino's privacy policy and may opt-out at anytime.