Subject archive for "machine-learning," page 23
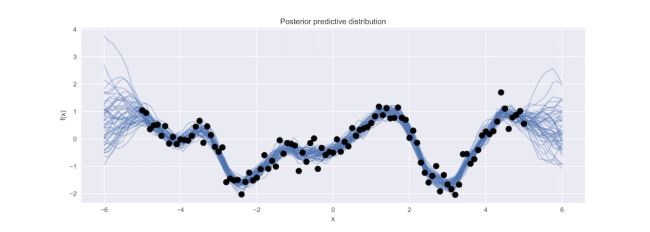
Fitting Gaussian Process Models in Python
A common applied statistics task involves building regression models to characterize non-linear relationships between variables. It is possible to fit such models by assuming a particular non-linear functional form, such as a sinusoidal, exponential, or polynomial function, to describe one variable's response to the variation in another. Unless this relationship is obvious from the outset, however, it involves possibly extensive model selection procedures to ensure the most appropriate model is retained. Alternatively, a non-parametric approach can be adopted by defining a set of knots across the variable space and use a spline or kernel regression to describe arbitrary non-linear relationships. However, knot layout procedures are somewhat ad hoc and can also involve variable selection. A third alternative is to adopt a Bayesian non-parametric strategy, and directly model the unknown underlying function. For this, we can employ Gaussian process models.
By Chris Fonnesbeck27 min read
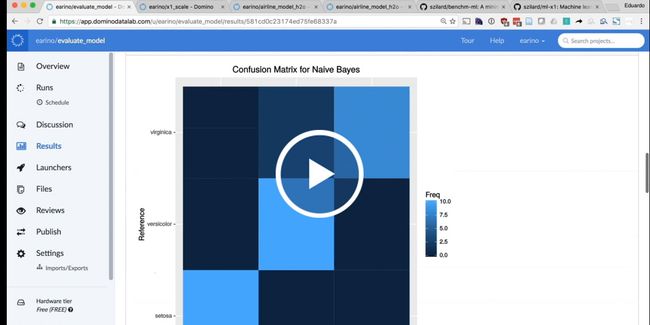
Exploring the Limits of Parallelized Machine Learning
This week, Domino’s Chief Data Scientist, Eduardo Ariño de la Rubia, presented a webinar: Machine Learning at Scale with Amazon's X1 Instance. If you missed the live webinar or would like to watch it again, you can find a recording below:
By Sheila Doshi1 min read
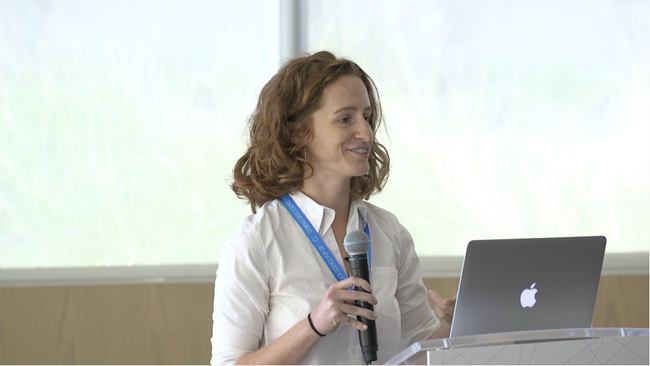
How Buzzfeed Uses Real-Time Machine Learning to Choose Their Viral Content
This talk took place at the Domino Data Science Pop-up in Los Angeles, CA on September 14, 2016.
By Sheila Doshi1 min read
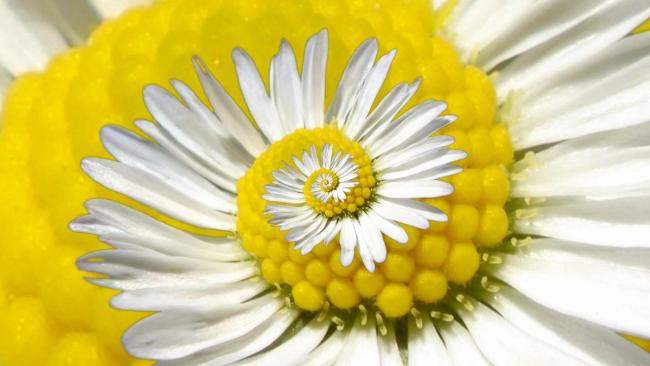
Wisdom From Machine Learning at Netflix
At Data By The Bay in May, we saw a great talk by Netflix's Justin Basilico: Recommendations for Building Machine Learning Software. Justin describes some principles for effectively developing machine learning algorithms and integrating them into software products.
By Nick Elprin3 min read
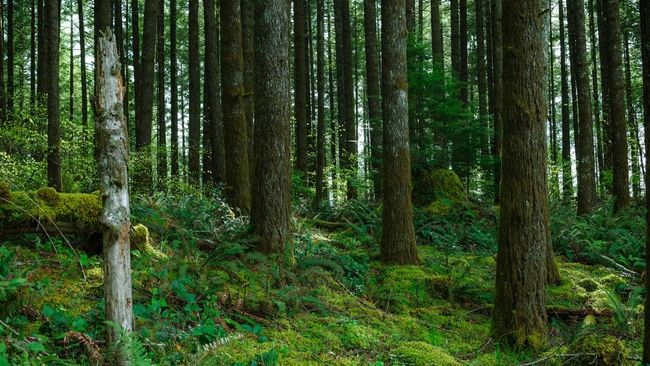
High-performance Computing with Amazon's X1 Instance - Part II
When you have at your disposal 128 cores and 2TB of RAM, it’s hard not to experiment and attempt to find ways to leverage the amount of power that is at your fingertips. We’re excited to remind our readers that we support Amazon’s X1 instances in Domino, you can do data science on machines with 128 cores and 2TB of RAM — with one click:
By Eduardo Ariño de la Rubia5 min read
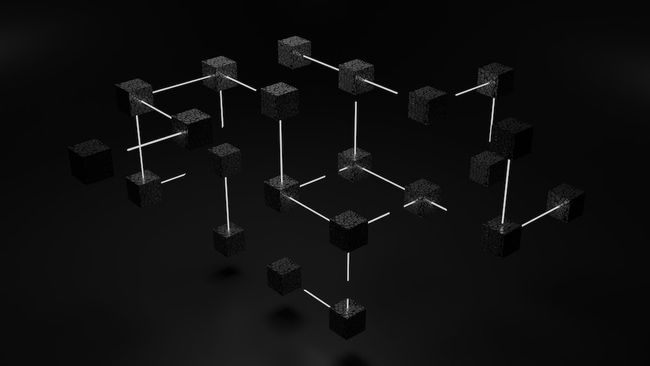
Using k-Nearest Neighbors (k-NN) in Production
What is k-Nearest Neighbors (k-NN)?
By Sheila Doshi1 min read
Subscribe to the Domino Newsletter
Receive data science tips and tutorials from leading Data Science leaders, right to your inbox.
By submitting this form you agree to receive communications from Domino related to products and services in accordance with Domino's privacy policy and may opt-out at anytime.