Subject archive for "perspective," page 9
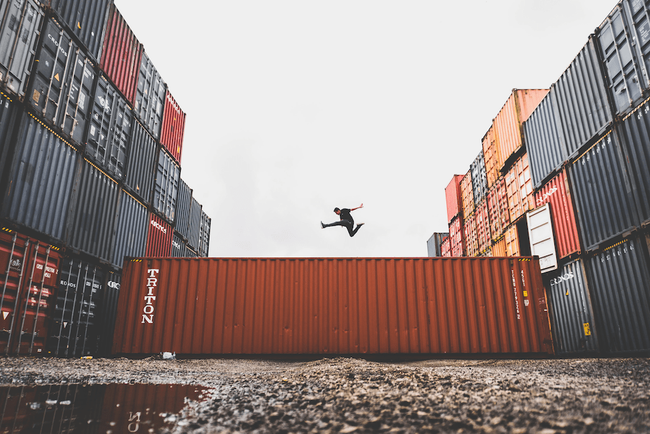
Why Kubernetes is Great for Data Science Workloads
Kubernetes is an open-source container orchestration system that is quickly becoming essential to IT departments as they move towards containerized applications and microservices. As powerful as Kubernetes is with general IT workloads, Kubernetes also offers unique advantages to support bursty data science workloads. With the help of containers, data scientists can make their modeling portable and reproducible, and massively scale these same, containerized machine learning models.
By Bob Laurent9 min read
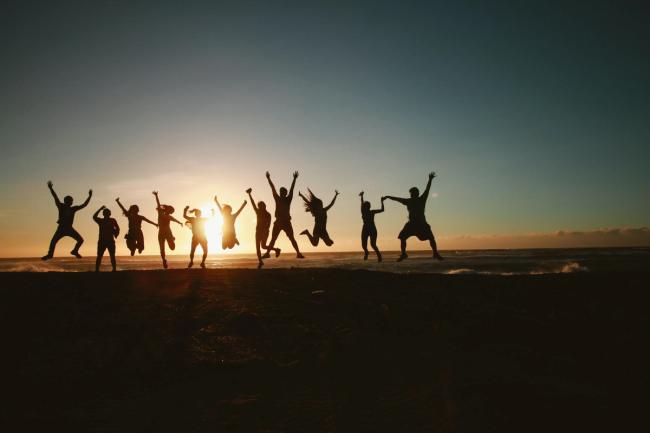
Getting data science to enterprise scale rapidly: A conversation with CSL Behring’s John K. Thompson
Data science’s impact has become increasingly clear in every industry as leading adopters raise the bar for personalized services and customer experiences. IDC’s recent survey on AI found that early adopters reported a 25 percent improvement in customer experience along with accelerated rates of innovation and higher competitiveness, among other benefits.
By Karina Babcock11 min read
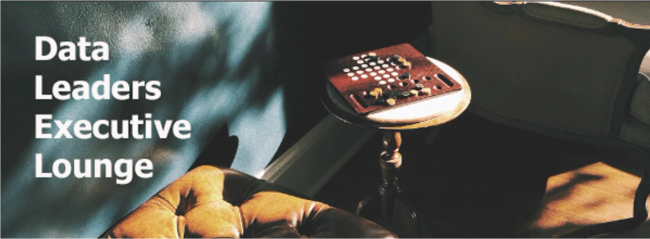
Creating a community of impactful data leaders during a lockdown
This article was originally published to LinkedIn. Thanks to Dan Harris for giving us permission to re-post it here.
By Dan Harris9 min read
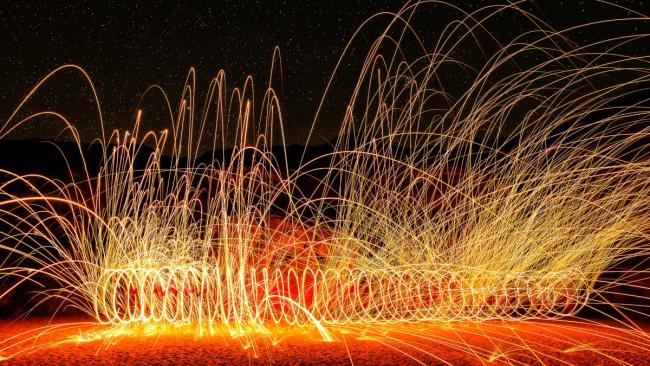
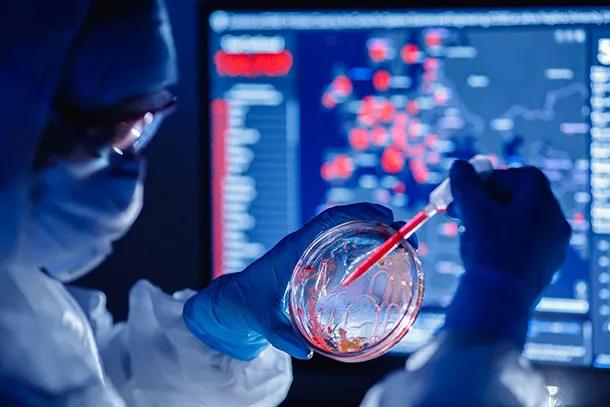
Life Sciences Teams: Avoid These Common Pitfalls on Your Path to the Cloud
Many life sciences organizations are in the midst of digital transformation efforts. A key component of this is moving existing on-premises IT platforms, statistical computing environments (SCEs), and associated programming languages and tools into the cloud. Research and Data Science functions represent low-hanging fruit for these cloud migrations because their workflows necessitate dynamic access to compute infrastructure and centralized access to diverse toolsets.
By Tim Hughes10 min read
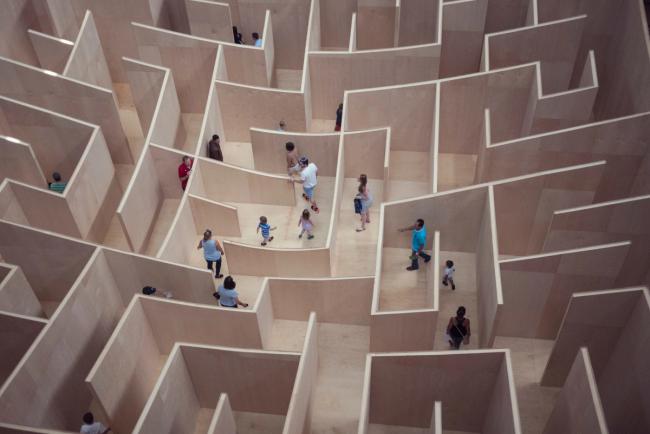
Why models fail to deliver value and what you can do about it
Building models requires a lot of time and effort. Data scientists can spend weeks just trying to find, capture and transform data into decent features for models, not to mention many cycles of training, tuning, and tweaking models so they’re performant.
By David Bloch9 min read
Subscribe to the Domino Newsletter
Receive data science tips and tutorials from leading Data Science leaders, right to your inbox.
By submitting this form you agree to receive communications from Domino related to products and services in accordance with Domino's privacy policy and may opt-out at anytime.