DataOps delivers data science value faster for Moneysupermarket
Harvinder Atwal2020-05-28 | 7 min read
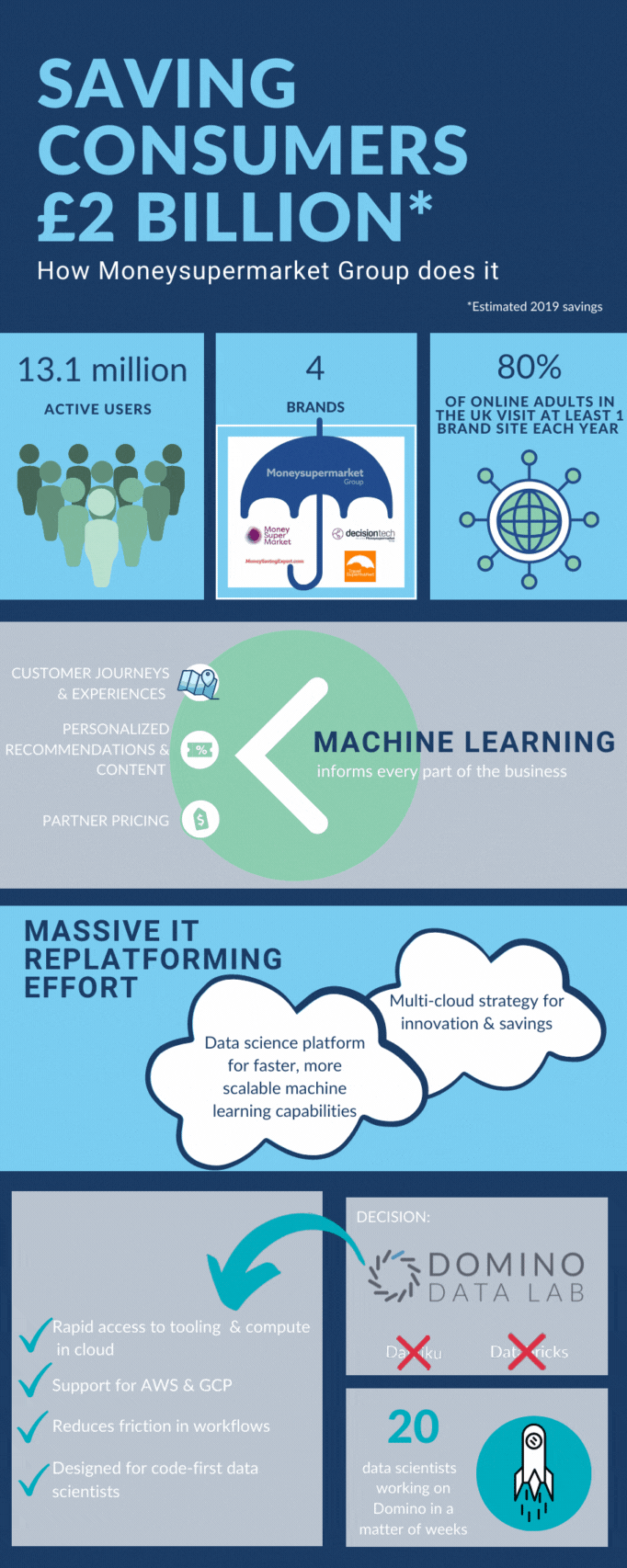
By Harvinder Atwal, Chief Data Officer, The Moneysupermarket Group on May 28, 2020 in Perspective
Editor’s note: This is part of a series of articles sharing best practices from companies on the road to becoming model-driven. Some articles will include information about their use of Domino.
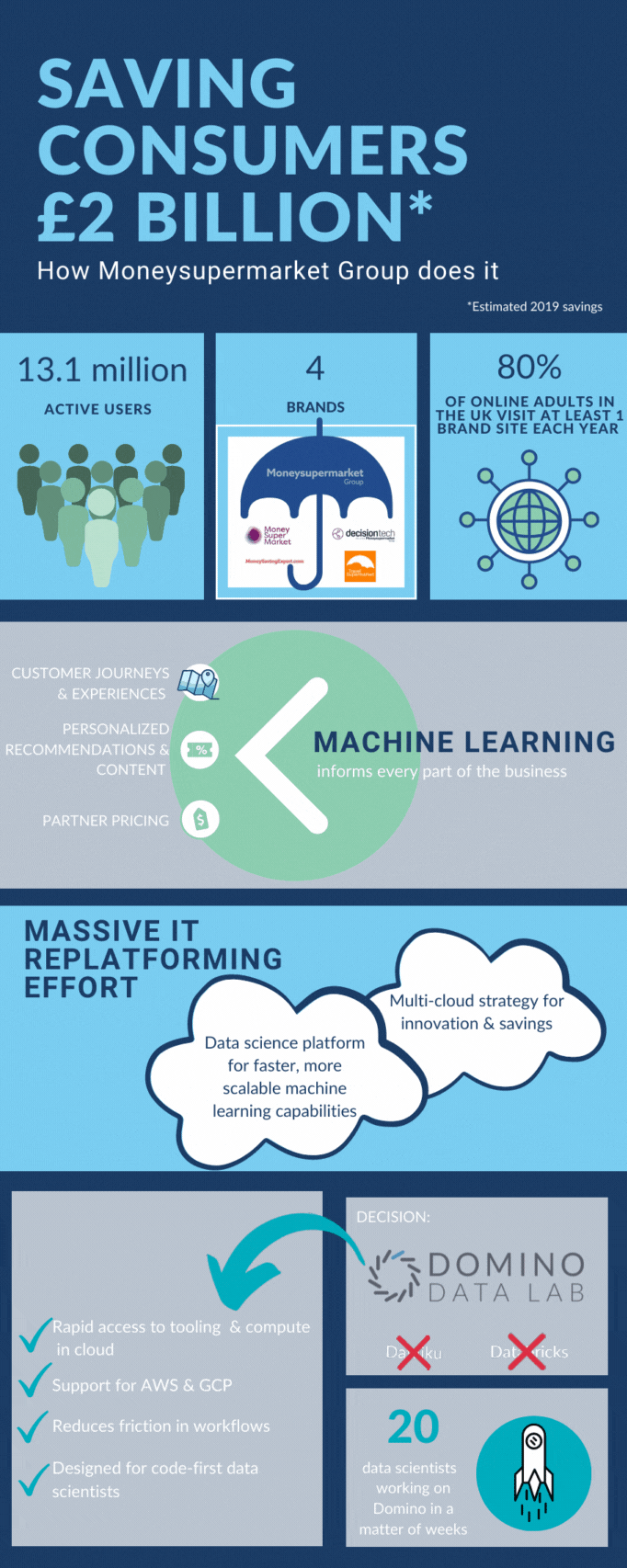
Several years ago, my team at Moneysupermarket stumbled on DataOps. By stumbled, I mean that we didn’t actively set out to implement the DataOps methodology, which had begun gaining traction as a way to operationalise and scale data science faster. Instead, we began applying best practices from software development, product management, and product engineering out of basic necessity.
What happened?
It started with a simple question: Could we use data science to personalise communications and content for customers based on their financial needs, risk profile, and attitudes?
Moneysupermarket’s primary mission is to help consumers save money on everything from insurance and credit cards to utilities and travel. Last year, we served more than 13 million active users and delivered an estimated £2 billion in annual savings to consumers through our four brands: MoneySuperMarket, TravelSupermarket, MoneySavingExpert, and Decision Tech.
We have thousands of products, but at the time, our marketing team used a one-size-fits-all approach for communicating with customers. We believed that personalisation was key to increasing revenue. For example, rather than sending one newsletter to all customers, the marketing team could use data science to tailor timing, language, and content, sending different variants of the newsletter to different types of customers.
Business stakeholders were interested in the concept, but to gain their full support and funding, we needed to show them results—and fast. We only had two months to prove that a model-driven personalisation approach was better than the hypothesis and rules-driven approach the stakeholders originally intended.
Our data science processes weren’t optimised for speed. This situation isn’t unusual. Most data science teams can likely point to bottlenecks in the data science lifecycle, such as an inability to quickly access the data, tools, and compute resources they need or rapidly deploy experiments and iterate with stakeholders. These bottlenecks prevent them from achieving the full return from their investments.
In fact, according to recent research from NewVantage Partners, just 7.3 percent of organisations say the state of their data and analytics are excellent.
We had to change how we worked.
We decided to draw from software development, product engineering, and product management best practices to rapidly create a minimum viable product for personalising communications. We looked to incorporate three fundamental approaches:
- Agile practices, especially when it came to collaborating with business stakeholders. We sat down with our marketing stakeholders to identify the ultimate objective (for example, was it to improve customer experience or increase revenue?), which hypotheses to test, and what data products would meet these objectives. By doing so, we could assure our final product would address their needs and gain their full backing. We also created fast feedback loops that enabled us to test the new models with Marketing so we could see what worked and what didn’t, and then quickly refine our models.
- Lean manufacturing principles to help eliminate waste and bottlenecks in the data science lifecycle, improve the quality of our final data products, and monitor data flows. We also looked to make data scientists more self-sufficient and ensure work was reproducible and reusable. For example, our Domino platform is a significant part of our DataOps ecosystem, helping us reduce waste and enforce better control as data scientists build and deploy models.
- DevOps culture, applying concepts like version control, configuration management, continuous integration, and continuous operations to develop and deploy models faster and more reliably.
Even we were surprised by the results.
By applying these practices (all of which are foundational to DataOps), we dramatically reduced how long it took to develop, test, and deploy new personalisation models. In three months, we were analysing experiments. Within six months, our new personalisation models were deployed and delivering value—much earlier than we had originally anticipated.
Today, rather than one newsletter, we have 1,400 variants, each tailored to the customer’s financial attitude and knowledge. Our models found, for example, that the use of positive sentiment and proper nouns increase conversions with customers who are more financially savvy and know what they want and need from our price comparison sites. In contrast, less financially savvy customers are more apt to respond to content that uses negative sentiment and modals like “could,” “should,” and “would.”
Since then, we’ve more formally adopted DataOps to scale our success.
This work set the foundation for our eventual DataOps journey, a journey that has enabled us to move quickly, using scalable and repeatable processes and highly optimised tools.
Through DataOps, we’ve cut weeks, months, and in a few cases, years off processes to enable Moneysupermarket to innovate faster and unlock new market opportunities.
We now have more than 50 models in production, informing every facet of our operations from optimising customer journeys and customer experiences, to personalising offers and content, to negotiating pricing with partners. It’s led to millions of pounds in additional revenue and enabled us to maintain our leadership position in a highly competitive marketplace.
DataOps requires a continuous improvement mindset based on gathering data and acting on feedback. We are constantly looking to increase our impact on customers and stakeholders by continually improving the speed at which we create and iterate data products.
Learn more:
- Read the Domino case study on Moneysupermarket.
- Read Harvinder’s interview with Computer Weekly on Moving to DataOps
- Read Harvinder’s book, Practical DataOps: Delivering Agile Data Science at Scale
RELATED TAGS
Subscribe to the Domino Newsletter
Receive data science tips and tutorials from leading Data Science leaders, right to your inbox.
By submitting this form you agree to receive communications from Domino related to products and services in accordance with Domino's privacy policy and may opt-out at anytime.